Telecom infrastructures are of vital importance to society. More and more applications depend on well-functioning, reliable and always available telecom services. The Dutch Radiocommunications Agency (Agentschap Telecom) oversees these in the Netherlands. The emergence of Artificial Intelligence (AI) applications has not only fundamentally changed the nature of the telecom sector but also the risks. In order to safeguard the proper functioning of the telecom infrastructure, adjustments are required in the relevant knowledge and in the approach to supervision policy.
Beginning 2020 I led a study to provide insight This report provides insight into how AI applications impact and endanger the telecom landscape and suggests how the Radiocommunications Agency can continue to maintain society’s trust in the telecom infrastructure.
What are the current and future risks of applying AI in the telecom sector?
Bearing in mind recent developments, a relevant description of AI is: using algorithms based on deep learning, and learning assisted by big data, to automate tasks that could formerly only be undertaken (properly) by humans. AI is expected to play an increasingly central role in telecom networks.
AI applications have specific characteristics that can pose risks for telecom infrastructures (the application level). Various AI applications interact with each other, with people, ‘normal’ automation and possibly the outside world. It is therefore important to assess how AI is applied in the telecom sector at a systemic level; that is to say looking at the effects and risks for the entire chain rather than AI applications in isolation. In particular, they include the ultimate use of these applications based on telecom infrastructures.
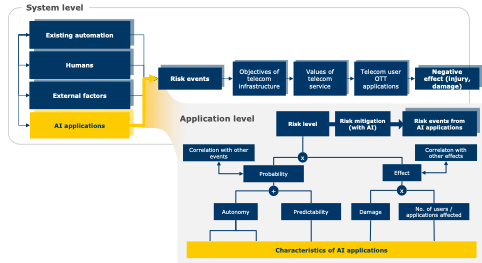
At application level, the extent of autonomous learning and operations, as well as the unpredictability, action framework and sphere of influence of AI applications determine the probability and impact of the additional risks. On top of the entire lifecycle of an AI application, including planning, data collection, training, testing, validation and operations, are notwithstanding the conventional risks relating to information security.
Although AI applications introduce new (types of) risks, ultimately they can add specific value to the process of mitigating risk.
How can the Dutch Radiocommunications Agency as supervisory and implementing organization mitigate these risks?
We recommend starting with tools at a systemic level. The Dutch Radiocommunications Agency could mitigate the risks of AI applications in the telecom sector by providing information and raising awareness, stipulating transparency, facilitating risk analysis and mitigation, as well as developing criteria and setting process requirements. There are specific tools for dealing with certain AI risk factors. At application level, more specific tools could be implemented: certification, auditing and maintaining particular types or aspects of AI could play a role. In a wider sense, there should be a social debate about the desirable level of telecom infrastructures’ provision.
What does the use of AI look like now and in the coming five years for the telecom sector and other sectors that use digital connectivity?
Most of the current AI applications focus on improving specific parameters. These are strictly defined applications such as optimising the parameters of a radio signal, power management or routing traffic through a network.
Looking at the coming five years, we see AI applications becoming more and more advanced. Several suppliers of telecom equipment share the view that AI will control the majority of functions in telecom networks. Although it is questionable whether this will be implemented (entirely) in five years, their vision is definitely one we can expect.
How do we weigh up the risks to the various interrelated aspects in a risk model for digital connectivity?
Certain characteristics of AI applications can pose additional risks for telecom infrastructures. These characteristics relate to the following aspects of AI:
- The extent of autonomous learning and implementation of AI. If this extent is considerable, the likelihood of risk events increases. A significant parameter is whether the AI application is controlled by people or by rules.
- The extent of the AI application’s predictability. If the models are non-deterministic or highly non-linear, it is more complicated to assess whether an application will work well in all situations. One influential factor is the type of data used and if it can be manipulated.
- The AI application’s action framework. If the AI application has a highly limited effect on telecom infrastructures, this restricts the impact of a risk event. An application with a wide operating framework has a potentially greater impact.
- The AI application’s sphere of influence. An application operating at a central level and controlling a telecom infrastructure is more prone to risk than an application that optimises a specific parameter at a low level.
The diagram below is an overview of the relevant aspects and their weighting. The scores can be combined under the “application level”.
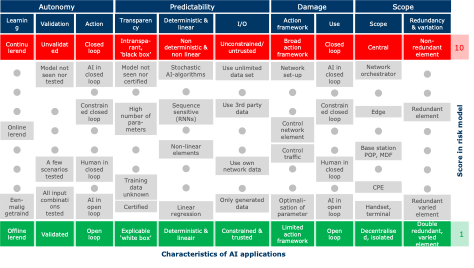
Considering the risks of AI applications in isolation paints a limited picture of the societal risks (as well as advantages) of implementing AI in telecom infrastructures. At the systemic level, the following factors affect risks:
- Interaction between AI applications and other systems.
- Replacing humans with AI. Having people carry out tasks involves risks, and these can be higher or lower with an AI application. This study does not chart the risks involved with human activities in telecom infrastructures. The model we present can be used to assess the risks of substituting with AI in order to inform the decision whether or not to implement a human-replacement AI application.
- Implement AI applications to mitigate risk. At a systemic level, AI applications can contribute to lowering the level of risk, for example through faster detection of problems or attacks, and by helping to find causes and solutions.
- Cyber (in)security of AI applications. AI applications are of course also subject to cyber threats and associated security risks. These risks may increase, because training AI applications involves bringing together large amounts of (sometimes sensitive) data.